Event Recap - Optimizing AI Agents: A Deep Dive into Fine-Tuning
General
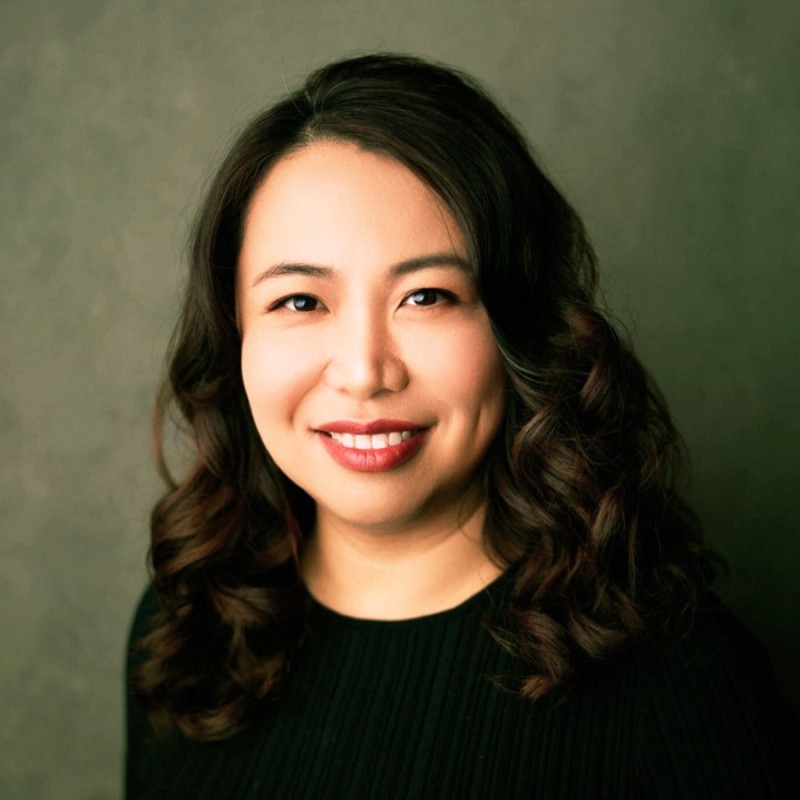
Catherine Qu
,
VP of Marketing
December 4, 2024
Download the presentation deck here.
Okareo hosted an event on optimizing AI agents and the importance of fine-tuning in collaboration with AICamp on December 4, 2024. Speakers included Matt Wyman, Co-Founder and CEO of Okareo and Boris Selitser, Co-Founder and CTO/CPO of Okareo. They shared their perspectives on fine-tuning and its practical applications in building and optimizing AI agents. Boris also stepped through how Okareo can help automate fine-tuning with synthetic data to boost results. Attendees gained valuable insights into the challenges and opportunities of fine-tuning, empowering them to make more informed decisions in their AI agent development journey.
Key Points from the Presentation:
AI agents combine knowledge, reasoning, tool use, and human input to interact with their environment and achieve goals. They learn from data, extract patterns, and make decisions based on their understanding of the world.
Fine-tuning is a technique used to adapt pre-trained models to specific tasks or domains. It involves adjusting the model's parameters to better align with the target task or domain.
Fine-tuning is particularly beneficial in situations where specialized agents are needed for specific tasks, where task-specific optimization is required, or where a domain-specific performance boost is desired.
However, fine-tuning may not be optimal in all cases. When general-purpose agents suffice, prompt engineering is effective, the latest model advances are desired, data is scarce, or open-source model management is complex, fine-tuning may not be necessary or feasible.
Key Takeaways:
Evaluation metrics are crucial for assessing agent performance and guiding fine-tuning efforts. Different metrics may be appropriate depending on the specific task or domain, and it is important to choose metrics that align with the desired outcomes.
Agent knowledge and retrieval mechanisms are sensitive to training data, and their optimization is essential for good performance. Fine-tuning can help improve the agent's ability to extract relevant information from the training data and use it effectively in decision-making.
Isolating key parts of the agent system and fine-tuning them individually can improve reliability and speed. By focusing on specific components of the agent, fine-tuning can be more targeted and efficient.
Synthetic data can be used to augment evaluation datasets and improve fine-tuning results. Synthetic data can be generated to simulate real-world conditions, providing a more comprehensive and diverse dataset for fine-tuning.
Conclusion:
The Okareo event provided valuable insights into the optimization of AI agents and the role of fine-tuning in achieving superior performance. As the field of AI continues to advance, fine-tuning and other optimization techniques will become even more critical for developing powerful and capable AI agents. By leveraging the latest research, best practices, and evaluation and fine-tuning platforms such as Okareo, organizations can harness the full potential of AI to drive innovation and solve complex problems.
Download the presentation deck here.
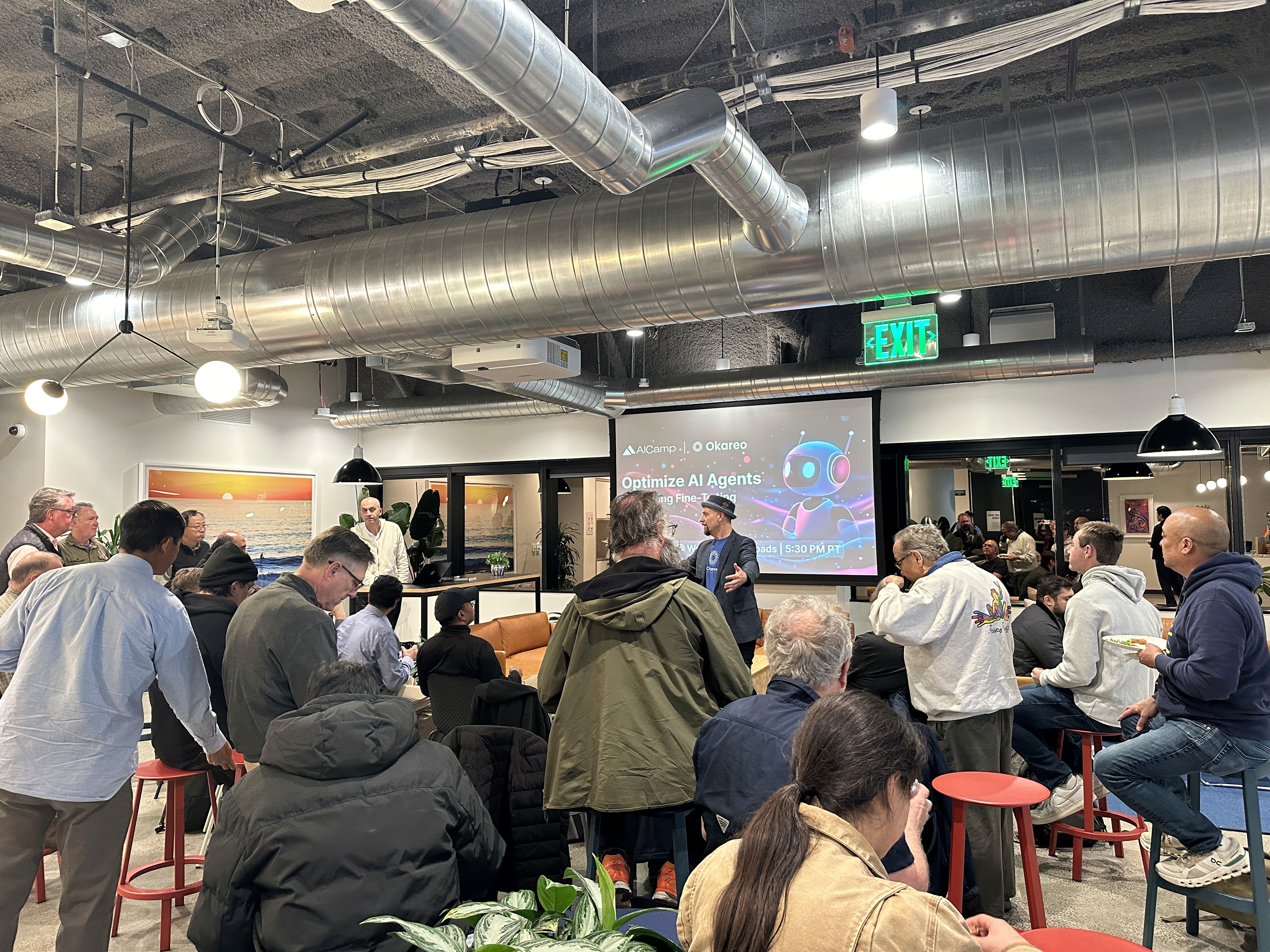

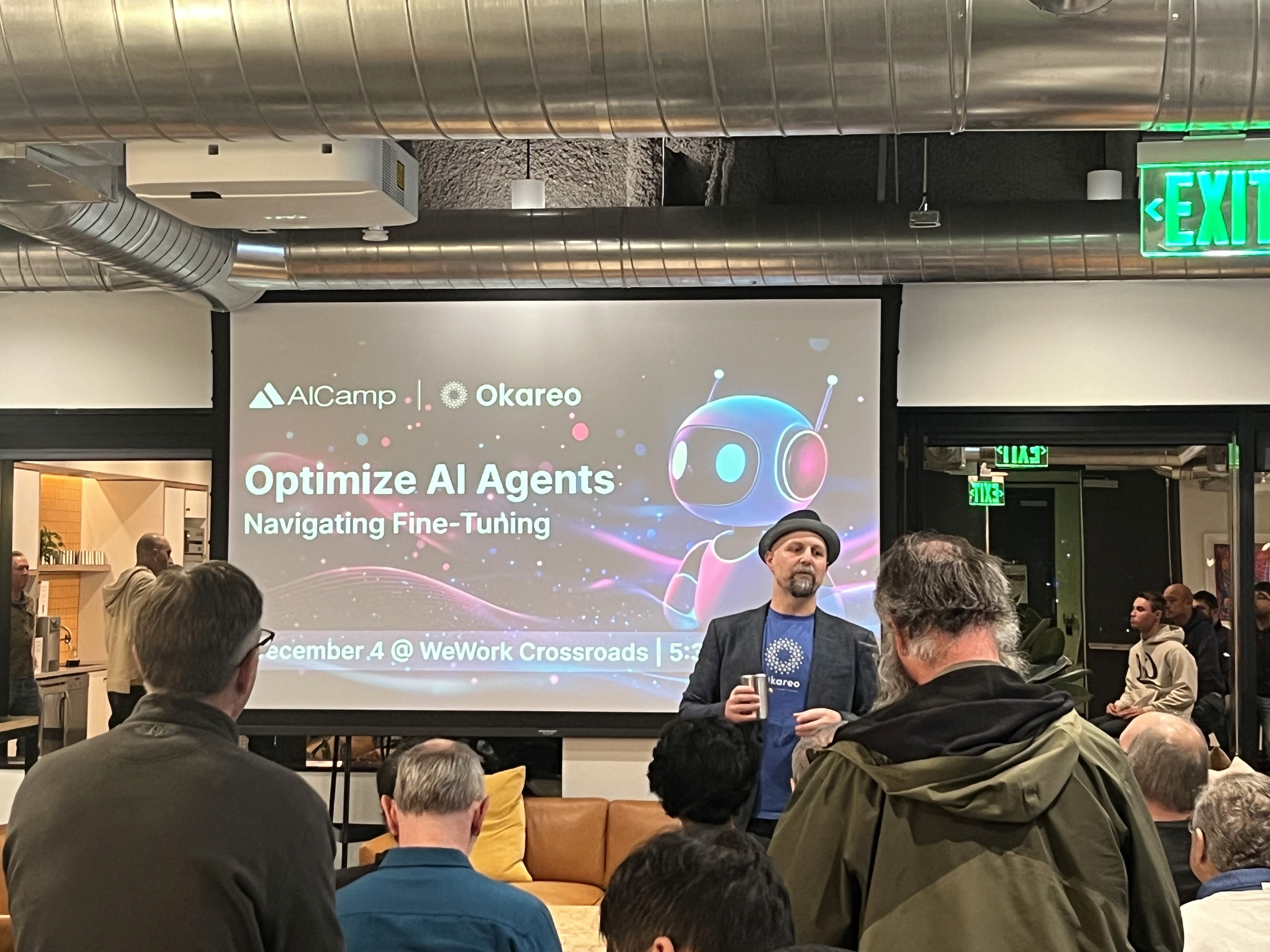

Download the presentation deck here.
Download the presentation deck here.
Okareo hosted an event on optimizing AI agents and the importance of fine-tuning in collaboration with AICamp on December 4, 2024. Speakers included Matt Wyman, Co-Founder and CEO of Okareo and Boris Selitser, Co-Founder and CTO/CPO of Okareo. They shared their perspectives on fine-tuning and its practical applications in building and optimizing AI agents. Boris also stepped through how Okareo can help automate fine-tuning with synthetic data to boost results. Attendees gained valuable insights into the challenges and opportunities of fine-tuning, empowering them to make more informed decisions in their AI agent development journey.
Key Points from the Presentation:
AI agents combine knowledge, reasoning, tool use, and human input to interact with their environment and achieve goals. They learn from data, extract patterns, and make decisions based on their understanding of the world.
Fine-tuning is a technique used to adapt pre-trained models to specific tasks or domains. It involves adjusting the model's parameters to better align with the target task or domain.
Fine-tuning is particularly beneficial in situations where specialized agents are needed for specific tasks, where task-specific optimization is required, or where a domain-specific performance boost is desired.
However, fine-tuning may not be optimal in all cases. When general-purpose agents suffice, prompt engineering is effective, the latest model advances are desired, data is scarce, or open-source model management is complex, fine-tuning may not be necessary or feasible.
Key Takeaways:
Evaluation metrics are crucial for assessing agent performance and guiding fine-tuning efforts. Different metrics may be appropriate depending on the specific task or domain, and it is important to choose metrics that align with the desired outcomes.
Agent knowledge and retrieval mechanisms are sensitive to training data, and their optimization is essential for good performance. Fine-tuning can help improve the agent's ability to extract relevant information from the training data and use it effectively in decision-making.
Isolating key parts of the agent system and fine-tuning them individually can improve reliability and speed. By focusing on specific components of the agent, fine-tuning can be more targeted and efficient.
Synthetic data can be used to augment evaluation datasets and improve fine-tuning results. Synthetic data can be generated to simulate real-world conditions, providing a more comprehensive and diverse dataset for fine-tuning.
Conclusion:
The Okareo event provided valuable insights into the optimization of AI agents and the role of fine-tuning in achieving superior performance. As the field of AI continues to advance, fine-tuning and other optimization techniques will become even more critical for developing powerful and capable AI agents. By leveraging the latest research, best practices, and evaluation and fine-tuning platforms such as Okareo, organizations can harness the full potential of AI to drive innovation and solve complex problems.
Download the presentation deck here.
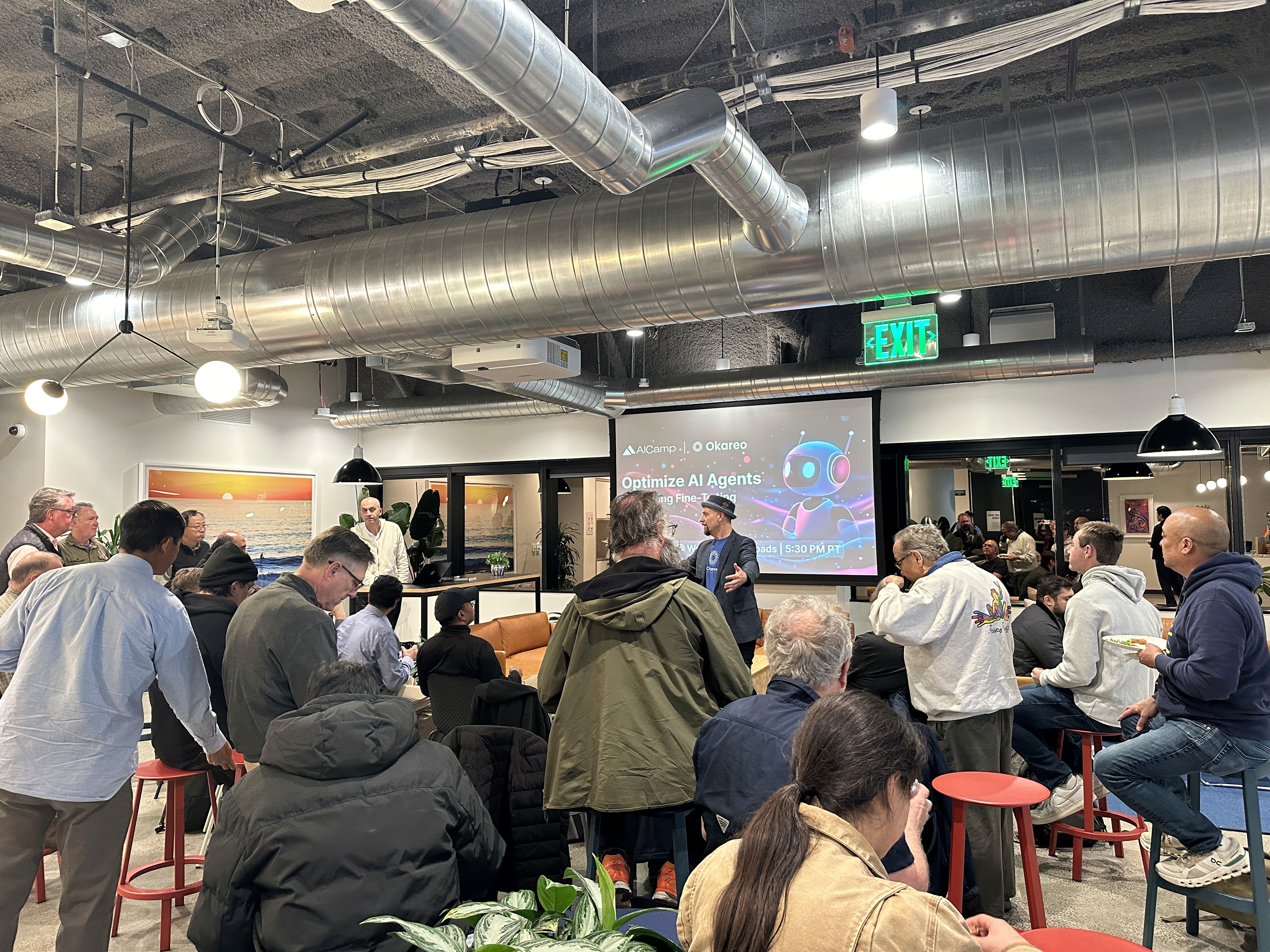

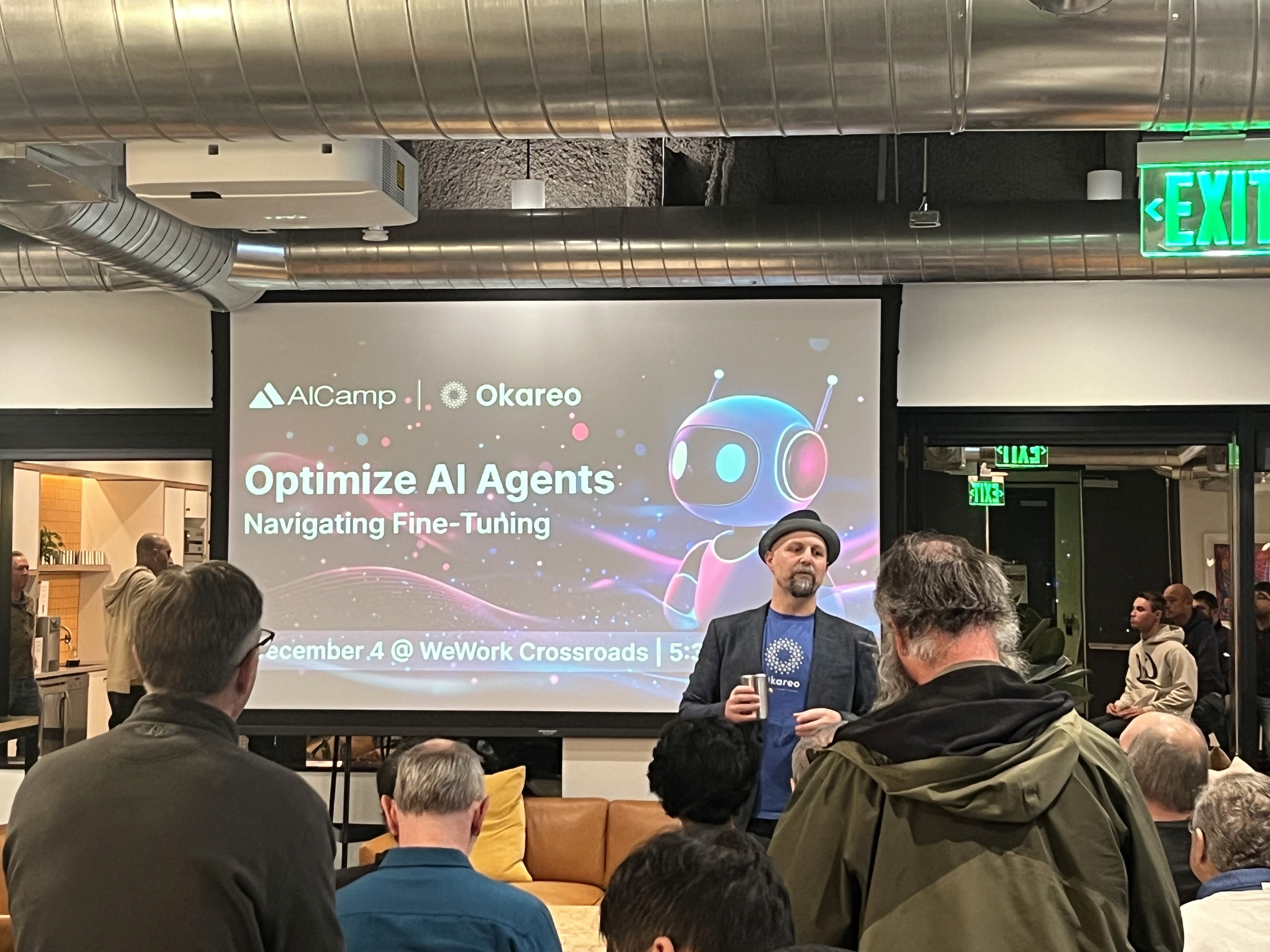

Download the presentation deck here.
Download the presentation deck here.
Okareo hosted an event on optimizing AI agents and the importance of fine-tuning in collaboration with AICamp on December 4, 2024. Speakers included Matt Wyman, Co-Founder and CEO of Okareo and Boris Selitser, Co-Founder and CTO/CPO of Okareo. They shared their perspectives on fine-tuning and its practical applications in building and optimizing AI agents. Boris also stepped through how Okareo can help automate fine-tuning with synthetic data to boost results. Attendees gained valuable insights into the challenges and opportunities of fine-tuning, empowering them to make more informed decisions in their AI agent development journey.
Key Points from the Presentation:
AI agents combine knowledge, reasoning, tool use, and human input to interact with their environment and achieve goals. They learn from data, extract patterns, and make decisions based on their understanding of the world.
Fine-tuning is a technique used to adapt pre-trained models to specific tasks or domains. It involves adjusting the model's parameters to better align with the target task or domain.
Fine-tuning is particularly beneficial in situations where specialized agents are needed for specific tasks, where task-specific optimization is required, or where a domain-specific performance boost is desired.
However, fine-tuning may not be optimal in all cases. When general-purpose agents suffice, prompt engineering is effective, the latest model advances are desired, data is scarce, or open-source model management is complex, fine-tuning may not be necessary or feasible.
Key Takeaways:
Evaluation metrics are crucial for assessing agent performance and guiding fine-tuning efforts. Different metrics may be appropriate depending on the specific task or domain, and it is important to choose metrics that align with the desired outcomes.
Agent knowledge and retrieval mechanisms are sensitive to training data, and their optimization is essential for good performance. Fine-tuning can help improve the agent's ability to extract relevant information from the training data and use it effectively in decision-making.
Isolating key parts of the agent system and fine-tuning them individually can improve reliability and speed. By focusing on specific components of the agent, fine-tuning can be more targeted and efficient.
Synthetic data can be used to augment evaluation datasets and improve fine-tuning results. Synthetic data can be generated to simulate real-world conditions, providing a more comprehensive and diverse dataset for fine-tuning.
Conclusion:
The Okareo event provided valuable insights into the optimization of AI agents and the role of fine-tuning in achieving superior performance. As the field of AI continues to advance, fine-tuning and other optimization techniques will become even more critical for developing powerful and capable AI agents. By leveraging the latest research, best practices, and evaluation and fine-tuning platforms such as Okareo, organizations can harness the full potential of AI to drive innovation and solve complex problems.
Download the presentation deck here.
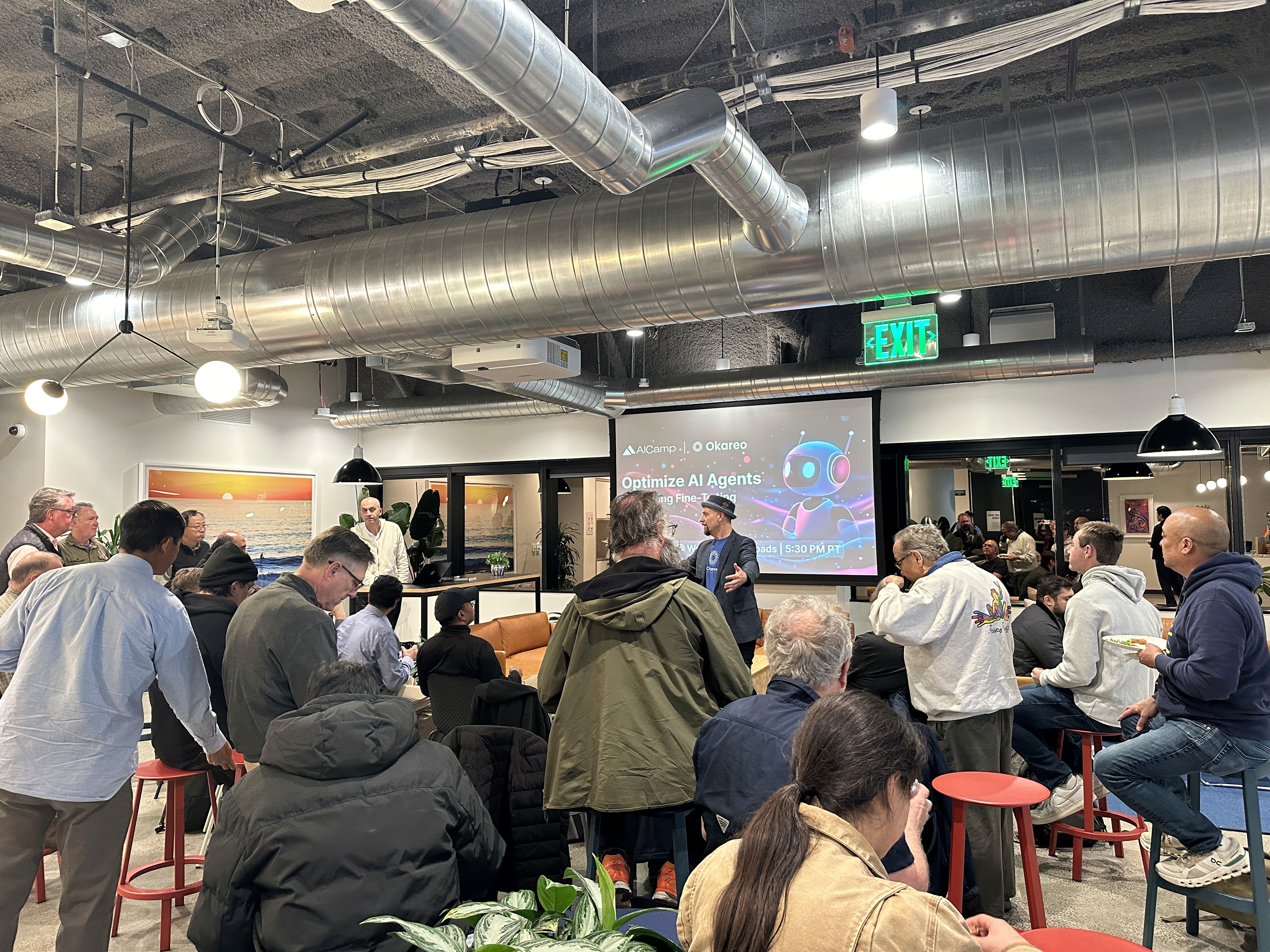

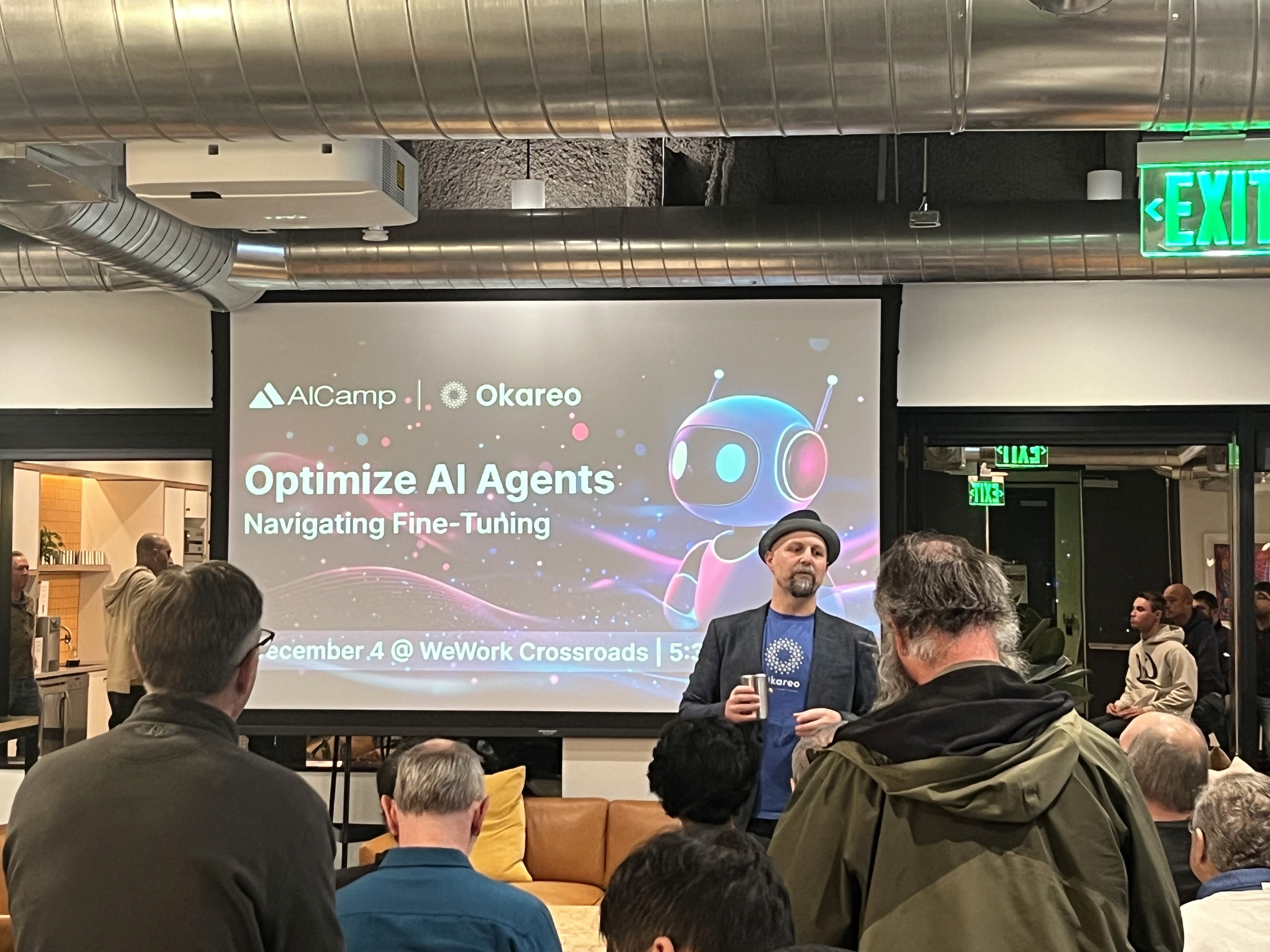

Download the presentation deck here.
Download the presentation deck here.
Okareo hosted an event on optimizing AI agents and the importance of fine-tuning in collaboration with AICamp on December 4, 2024. Speakers included Matt Wyman, Co-Founder and CEO of Okareo and Boris Selitser, Co-Founder and CTO/CPO of Okareo. They shared their perspectives on fine-tuning and its practical applications in building and optimizing AI agents. Boris also stepped through how Okareo can help automate fine-tuning with synthetic data to boost results. Attendees gained valuable insights into the challenges and opportunities of fine-tuning, empowering them to make more informed decisions in their AI agent development journey.
Key Points from the Presentation:
AI agents combine knowledge, reasoning, tool use, and human input to interact with their environment and achieve goals. They learn from data, extract patterns, and make decisions based on their understanding of the world.
Fine-tuning is a technique used to adapt pre-trained models to specific tasks or domains. It involves adjusting the model's parameters to better align with the target task or domain.
Fine-tuning is particularly beneficial in situations where specialized agents are needed for specific tasks, where task-specific optimization is required, or where a domain-specific performance boost is desired.
However, fine-tuning may not be optimal in all cases. When general-purpose agents suffice, prompt engineering is effective, the latest model advances are desired, data is scarce, or open-source model management is complex, fine-tuning may not be necessary or feasible.
Key Takeaways:
Evaluation metrics are crucial for assessing agent performance and guiding fine-tuning efforts. Different metrics may be appropriate depending on the specific task or domain, and it is important to choose metrics that align with the desired outcomes.
Agent knowledge and retrieval mechanisms are sensitive to training data, and their optimization is essential for good performance. Fine-tuning can help improve the agent's ability to extract relevant information from the training data and use it effectively in decision-making.
Isolating key parts of the agent system and fine-tuning them individually can improve reliability and speed. By focusing on specific components of the agent, fine-tuning can be more targeted and efficient.
Synthetic data can be used to augment evaluation datasets and improve fine-tuning results. Synthetic data can be generated to simulate real-world conditions, providing a more comprehensive and diverse dataset for fine-tuning.
Conclusion:
The Okareo event provided valuable insights into the optimization of AI agents and the role of fine-tuning in achieving superior performance. As the field of AI continues to advance, fine-tuning and other optimization techniques will become even more critical for developing powerful and capable AI agents. By leveraging the latest research, best practices, and evaluation and fine-tuning platforms such as Okareo, organizations can harness the full potential of AI to drive innovation and solve complex problems.
Download the presentation deck here.
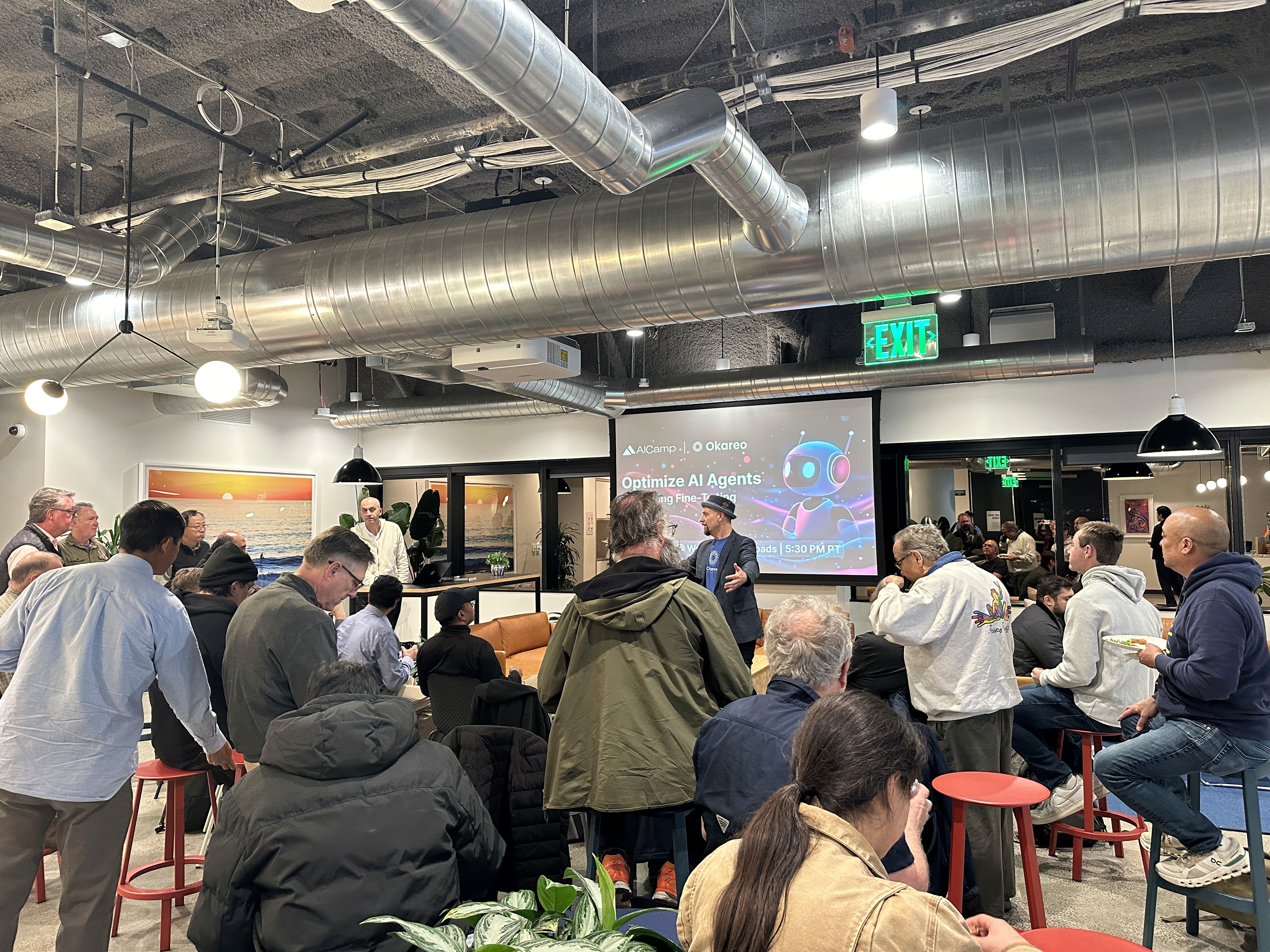

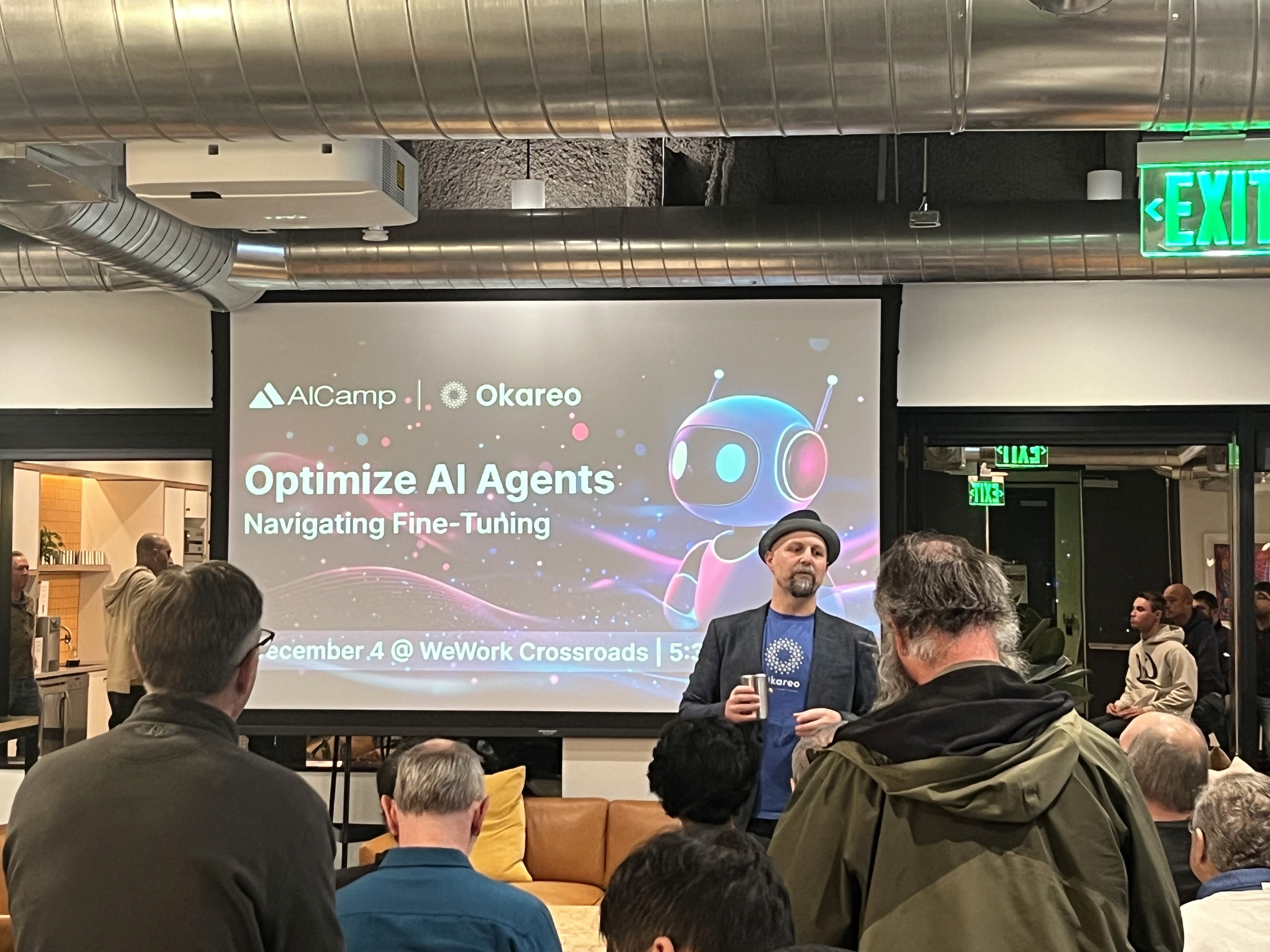

Download the presentation deck here.